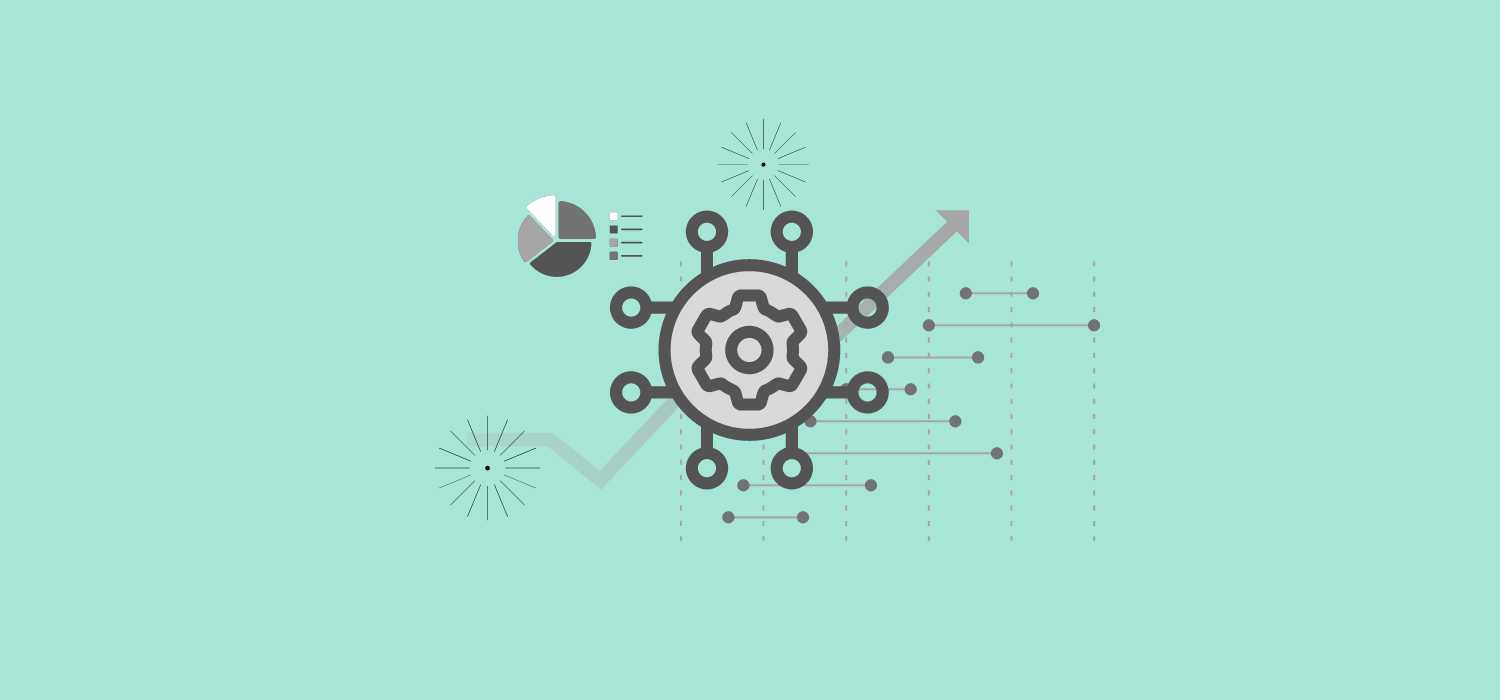
At their core, marketing attribution models are the method by which value is ascribed to a marketing channel. The optimal marketing attribution approach will depend largely on the data maturity of a business and its marketing mix: digital only, out of home, TV, etc.
Many marketers will be familiar with the last touch model wherein the full value of a transaction is attributed to the touchpoint which contains the conversion. This can be an effective method if the customer journey is simple but undervalue marketing channels that drive awareness. Last touch is an example of an arbitrary attribution model where the value of a touchpoint is determined by a simple heuristic (last touch, first touch, linear, time decay, etc).
Data driven attribution, however, is typically the optimal approach for digital attribution as it considers the full customer journey and attributes value to each touchpoint and does not assign the value arbitrarily, but rather, using mathematical models (such as Shapley or Markov).
Please note, not covered by this blog is marketing mix modelling (MMM). MMM is not limited to digital marketing alone, but also incorporates offline & out-of-home marketing.
MMM models are most useful when looking at optimisation between channels (e.g., should I spend more in Paid Search vs. TV) and understanding brand impact. However, it is not so useful for campaign optimisation as the level of granularity is limited.
An overview of the most common marketing attribution models:
1. Last click / last touch attribution: Gives all the credit to the last touchpoint that a user had before converting or taking a desired action
- Pros:
- Simple and easy to implement.
- Provides insight into the final push that led to a conversion.
- Cost-effective since it requires minimal data and analysis.
- Cons:
- Ignores earlier touchpoints, potentially undervaluing the impact of earlier marketing efforts.
- Offers a limited view of customer behaviour and preferences.
- Not an accurate representation of effectiveness of each marketing touchpoint.
- May encourage short-term thinking since it only focuses on the last touchpoint, potentially undervaluing the impact of longer-term marketing efforts that may have occurred earlier in the marketing journey.
2. First click / first touch attribution: Gives all the credit to the first touchpoint that a user had before converting or taking a desired action
- Pros:
- Useful for measuring the impact of top of funnel activities such as brand awareness campaigns
- Provides insight into the initial touchpoint that attracted the customer’s interest which can help organisations understand how to better engage potential customers
- Provides a starting point for analysing the customer journey
- Cons:
- Ignores later touchpoints in the customer journey, potentially undervaluing the impact of later marketing efforts.
- May not reflect actual customer behaviour as it gives full credit to the initial touchpoint even if the customer has multiple other touchpoints before converting.
- May undervalue bottom of funnel tactics, such as retargeting activites.
3. Linear attribution: Assigns equal credit to each touchpoint that a user had before converting or taking a desired action
- Pros:
- All touchpoints are considered.
- Demonstrates how each touchpoint has value.
- Provides a more comprehensive view of the customer journey compared with approaches such as last-click or first-click attribution.
- Encourages a more balanced approach when analysing marketing efforts
- Cons:
- Does not consider the varying impact of each touchpoint in the customer journey, likely undervaluing or overvaluing certain touchpoints.
- May overvalue middle of the funnel efforts.
- Assumes that each touchpoint is equally important in the customer journey which may not reflect actual customer behaviour.
4. Position-based / U-shaped attribution: Gives more credit to the touchpoints that occur at the beginning and end of a customer journey and distributes the remaining credit equally among the touchpoints in between
- Pros:
- Can help businesses optimise their marketing strategy to better engage customers at the first and last touchpoint in their journey which are often deemed crucial.
- Cons:
- Assumes that the first and last touchpoints are the most important which may not necessarily reflect actual customer behaviour.
- May undervalue significant touchpoints in the middle of the customer journey.
5. Time decay attribution: Gives more credit to the touchpoints that occur closest to the time of conversion and assigns less credit to the touchpoints that occurred earlier in the customer journey
- Pros:
- Reflects the impact of recent touchpoints which are often influential in driving a conversion.
- Cons:
- Assumes that touchpoints that occur closer to the time of conversion are the most important which may not always be the case.
- Can be less suitable for shorter sales cycles.
- Undervalues initial and middle touches, overlooking touchpoints that captured initial interest or were potentially crucial in influencing the customer’s eventual decision to convert.
6. Custom / Data-driven / algorithmic attribution: Uses machine learning to analyse the performance of each touchpoint in a customer journey and assigns credit accordingly
- Pros:
- Allows you control for how much value is allocated.
- Provides an objective view of the customer journey by analysing data, rather than relying on opinions or assumptions.
- Better reflects the behaviour of an organisation’s unique set customers, rather than making assumptions about their behaviour.
- Considers all touchpoints in the customer journey, providing a more complete view of how customers interact with the business.
- Helps businesses identify touchpoints that are most effective at driving conversions, allowing them to focus their marketing efforts on there.
- Part of an iterative process, so models improve as they develop in terms of the data sources being considered and the quality of data utilised.
- Cons:
- Requires effort to build and develop a data driven attribution model.
- Effort may be perceived as ‘front-loaded’, so it may be difficult for some organisations to make the first step.
- Tends to work best with larger amounts of data which may be a challenge for smaller businesses with limited amounts of data or the inexperience to retreive their data
- Can be complex and time-consuming to implement for some organisations, as it requires significant expertise in data and analytics.
- Requires effort to build and develop a data driven attribution model.
Why data-driven attribution may be the best marketing attribution model for your organisation:
- Data-driven attribution provides a full view of online journeys (offline journeys can be incorporated into analysis using probabilistic techniques)
- More accurate and objective: data-driven attribution uses machine learning to analyse a vast amounts of data and identify the most impactful touchpoints in the customer journey and provide a more accurate view of the value of each marketing channel (especially in comparison to attribution models that favour one channel or assign weightings to particular channels).
- Customisable: data-driven attribution can be tailored to suit the specific needs and goals of a business, allowing them to optimise attribution modelling based on the unique nature of their customer journeys, their marketing strategies and their circumstances.
- The big picture: data-driven attribution provides a full view of online and offline customer journeys, whether you are an awareness driver, action driver, or conversion driver.
- Data-driven attribution allows teams to understand the impact of their marketing activity wherever their role in the marketing funnel – whether they are an awareness driver, action driver or conversion driver – users have a complete understanding of the value of their channels without aspects being overlooked arbitrarily.
- Continuous improvement: data-driven attribution is an iterative process that gets better over time as more data is collected and analysed, providing organisations with increasingly accurate insights from an increasingly robust model
Does marketing attribution have any limitations?
Marketing attribution, while a valuable approach, does have certain limitations that should be considered. Some of the key limitations include:
- Lack of visibility into offline interactions: Attribution models are typically designed for digital marketing channels, where customer interactions can be easily tracked and measured. However, they may not capture the full picture when it comes to offline interactions, such as in-store visits or word-of-mouth referrals, which can play a significant role in customer decision-making.
- Incomplete data or data quality issues (most notably due to cookie consent): Accurate attribution requires reliable and comprehensive data on customer interactions. However, data gaps, measurement errors, or inconsistencies can impact the accuracy of attribution models, leading to biased or incomplete results.
- Timeframe and lag effects: Attribution models generally focus on short-term effects and immediate conversions. They may not adequately account for longer-term effects and the lag between marketing activities and desired outcomes, which can vary across different industries and customer segments.
Considering these limitations, it’s important to approach marketing attribution as a tool for insights and optimisation rather than a definitive measure of marketing effectiveness. It should be complemented with other analytical approaches and market research to gain a more comprehensive understanding of the overall impact of marketing efforts.
For example, marketing mix modelling (MMM) and marketing attribution can work in tandem to provide a more comprehensive understanding of marketing effectiveness. While attribution models focus on individual touchpoints and their contributions, MMM takes a holistic view by considering the overall impact of marketing inputs.
By combining the two approaches, marketers can gain valuable insights into the synergistic effects of different marketing channels and tactics. MMM can help validate and refine attribution results by incorporating broader market dynamics, external factors, and long-term effects. It can provide a more complete picture of how different marketing elements work together to drive business outcomes.
By leveraging both attribution and MMM, marketers can make informed decisions about resource allocation, campaign optimisation, and strategic planning, maximising the effectiveness of their marketing efforts. The integration of these approaches allows for a more nuanced and data-driven understanding of the complex relationship between marketing activities and business performance.
To find out how Lynchpin can help
About the author
Sam Clements
Sam has a degree in Natural Science and a master’s degree in Renewable Energy Engineering. As Data Science Manager at Lynchpin, Sam works on a broad range of projects across industries including Travel, Financial Services and Pharmaceuticals.
Specialising in marketing attribution, Sam utilises a range of languages (Python, R), web analytics platforms (Adobe and Google Analytics), cloud platforms (AWS, GCP), and reporting tools (Tableau, Power BI) to develop bespoke end-to-end models covering the mapping of customer journeys, spend optimisation & scenario planning.